NVIDIA’s DGX-2: Sixteen Tesla V100s, 30 TB of NVMe, only $400K
by Ian Cutress on March 27, 2018 2:00 PM EST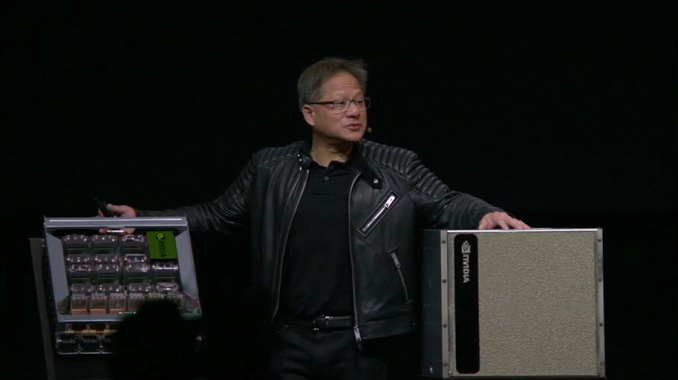
Ever wondered why the consumer GPU market is not getting much love from NVIDIA’s Volta architecture yet? This is a minefield of a question, nuanced by many different viewpoints and angles – even asking the question will poke the proverbial hornet nest inside my own mind of different possibilities. Here is one angle to consider: NVIDIA is currently loving the data center, and the deep learning market, and making money hand-over-fist. The Volta architecture, with CUDA Tensor cores, is unleashing high performance to these markets, and the customers are willing to pay for it. So introduce the latest monster from NVIDIA: the DGX-2.
DGX-2 builds upon DGX-1 in several ways. Firstly, it introduces NVIDIA’s new NVSwitch, enabling 300 GB/s chip-to-chip communication at 12 times the speed of PCIe. This, with NVLink2, enables sixteen GPUs to be grouped together in a single system, for a total bandwidth going beyond 14 TB/s. Add in a pair of Xeon CPUs, 1.5 TB of memory, and 30 TB of NVMe storage, and we get a system that consumes 10 kW, weighs 350 lbs, but offers easily double the performance of the DGX-1. NVIDIA likes to tout that this means it offers a total of ~2 PFLOPs of compute performance in a single system, when using the tensor cores.
NVIDIA DGX Series (with Volta) | ||
DGX-2 | DGX-1 | |
CPUs | 2 x Intel Xeon Platinum |
2 x Intel Xeon E5-2600 v4 |
GPUs | 16 x NVIDIA Tesla V100 32GB HBM2 |
8 x NVIDIA Tesla V100 16 GB HBM2 |
System Memory | Up to 1.5 TB DDR4 | Up to 0.5 TB DDR4 |
GPU Memory | 512 GB HBM2 (16 x 32 GB) |
256 GB HBM (8 x 32 GB) |
Storage | 30 TB NVMe Up to 60 TB |
4 x 1.92 TB NVMe |
Networking | 8 x Infiniband or 8 x 100 GbE |
4 x IB + 2 x 10 GbE |
Power | 10 kW | 3.5 kW |
Size | 350 lbs | 134 lbs |
GPU Throughput | Tensor: 1920 TFLOPs FP16: 480 TFLOPs FP32: 240 TFLOPs FP64: 120 TFLOPs |
Tensor: 960 TFLOPs FP16: 240 TFLOPs FP32: 120 TFLOPs FP64: 60 TFLOPs |
Cost | $399,000 | $149,000 |
NVIDIA’s overall topology relies on a dual stacked system. The high level concept photo provided indicates that there are actually 12 NVSwitches (216 ports) in the system in order to maximize the amount of bandwidth available between the GPUs. With 6 ports per Tesla V100 GPU, each running in the larger 32GB of HBM2 configuration, this means that the Teslas alone would be taking up 96 of those ports if NVIDIA has them fully wired up to maximize individual GPU bandwidth within the topology.
AlexNET, the network that 'started' the latest machine learning revolution, now takes 18 minutes
Notably here, the topology of the DGX-2 means that all 16 GPUs are able to pool their memory into a unified memory space, though with the usual tradeoffs involved if going off-chip. Not unlike the Tesla V100 memory capacity increase then, one of NVIDIA’s goals here is to build a system that can keep in-memory workloads that would be too large for an 8 GPU cluster. Providing one such example, NVIDIA is saying that the DGX-2 is able to complete the training process for FAIRSEQ – a neural network model for language translation – 10x faster than a DGX-1 system, bringing it down to less than two days total rather than 15.
Otherwise, similar to its DGX-1 counterpart, the DGX-2 is designed to be a powerful server in its own right. Exact specifications are still TBD, but NVIDIA has already told us that it’s based around a pair of Xeon Platinum CPUs, which in turn can be paired with up to 1.5TB of RAM. On the storage side the DGX-2 comes with 30TB of NVMe-based solid state storage, which can be further expanded to 60TB. And for clustering or further inter-system communications, it also offers InfiniBand and 100GigE connectivity, up to eight of them.
The new NVSwitches means that the PCIe lanes of the CPUs can be redirected elsewhere, most notably towards storage and networking connectivity.
Ultimately the DGX-2 is being pitched at an even higher-end segment of the deep-learning market than the DGX-1 is. Pricing for the system runs at $400k, rather than the $150k for the original DGX-1. For more than double the money, the user gets Xeon Platinums (rather than v4), double the V100 GPUs each with double the HBM2, triple the DRAM, and 15x the NVMe storage by default.
NVIDIA has stated that DGX-2 is already certified for the major cloud providers.
Related Reading
- Big Volta Comes to Quadro: NVIDIA Announces Quadro GV100
- The NVIDIA GTC 2018 Keynote Live Blog
- NVIDIA Develops NVLink Switch: NVSwitch, 18 Ports For DGX-2 & More
- NVIDIA Volta Unveiled: GV100 GPU and Tesla V100 Accelerator Announced
- NVIDIA Ships First Volta-based DGX Systems
- NVIDIA Unveils the DGX-1 HPC Server: 8 Teslas, 3U, Q2 2016
28 Comments
View All Comments
mode_13h - Monday, April 2, 2018 - link
No. Rack space isn't *that* valuable, especially when you consider the energy efficiency penalty of all the NVSwitches.eSyr - Wednesday, March 28, 2018 - link
One of the selling points there that DGX-2 equips V100s with 32 GB of HBM instead of 16 GB. The other one is that it employs much faster fabric. These two could drive significant improvement in performance on some workloads that has memory or bandwidth bottlenecks on DGX-1.mode_13h - Monday, April 2, 2018 - link
No, the memory increase is across-the-board. So, DGX-1 will also now ship with 32 GB per V100.Yojimbo - Friday, March 30, 2018 - link
It depends on the workload. Compute performance is useless if it is heavily constrained by communications bandwidth. The DGX-2 allows every GPU in the node direct memory access at 300 GB/s to all HBM memory in the node (the memory on its own package is still 900 GB/s of course). That's one big pool of 512 GB. Two DGX-1s linked via Infiniband or Ethernet would have two pools of 256 GB (after upgrading to 32 GB V100s). Within one pool (node) each GPU would enjoy only 50 or 100 GB/s of direct memory access bandwidth and between pools the latency for memory operations would be much higher and the bandwidth would be lower than in the DGX-2 where the operations are carried out within one pool.mode_13h - Monday, April 2, 2018 - link
You're not wrong. The point of this thing is mainly to efficiently scale up neural network training to larger models than you could fit on the 8 V100's that a DGX-1 can host.If you don't need more than 8x V100s in a single box, then you probably wouldn't be buying this.
eSyr - Wednesday, March 28, 2018 - link
DGX-1 has only 8×16 == 128 GB of GPU memory.frenchy_2001 - Wednesday, March 28, 2018 - link
Not anymore. It may take some time to trickle to the OEMs, but all V100 are now fitted with 32GB of HBM2 and NV will keep selling DGX1.So, older DGX1 will hve 16GB/GPU, but newer ones, comparable to the DGX2, will have 32GB.
Biggest difference will be density and fabric. DGX2 allows all 16 GPUs to share their memory.
Ferrynthia - Sunday, April 1, 2018 - link
Guys, i know, you love vulgar girlsWhat about online communication with them without limits? Here http://lonaism.ga you can find horny real girls from different countries.